Content from Introduction
Last updated on 2024-09-20 | Edit this page
Estimated time: 12 minutes
Overview
Questions
- How do we define software in research?
- What is the classical software lifecycle?
- What are the FAIR principles applied to research software?
Objectives
- Understand the definition of software in research.
- Understand the FAIR principles as applied to research software.
- Get to know (briefly) the software lifecycle.
What is a software in academia?
It is not always easy to define what constitutes software in a research setting. The size of projects can vary from a small script of a few dozens of lines to a massive project with millions of lines. If you are interested in a discussion around a research software definition a good starting point is Defining Research Software: a controversial discussion. An abbreviated summary of this paper and a pragmatic definition we use as a basis for this course is…
Key Points
Research Software includes source code files, algorithms, scripts, computational workflows and executables that were created during the research process or for a research purpose. Software components (e.g., operating systems, libraries, dependencies, packages, scripts,etc.) that are used for research but were not created during or with a clear research intent should be considered software in research and not Research Software. This differentiation may vary between disciplines.
Reminder: The FAIR principles applied to research software
The FAIR principles (Findable, Accessible, Interoperable and Reusable) were originally designed for research data in order to “enhance their re-usability” (see Wilkinson et al (2016)). In this seminal paper it was made clear that while data was a central aspect of research, the principles should also apply to algorithms, tools and workflows that led to the production of that data. Few years later, in 2022, a set of recommendation was published (Chue Hon et al. (2022); Barker et al. (2022)) in order to apply these FAIR principle to research software. An overview of the FAIR principles adapted to research software are that…
Key Points
Findable: Software, and its associated metadata, is easy for both humans and machines to find.
Accessible: Software, and its metadata, is retrievable via standardised protocols.
Interoperable: Software interoperates with other software by exchanging data and/or metadata, and/or through interaction via Application Programming Interfaces (APIs), described through standards.
Reusable: Software is both usable (can be executed) and reusable (can be understood, modified, built upon, or incorporated into other software).
The software lifecycle
A traditional software lifecycle (in a non-academic setup) involves the following phases:
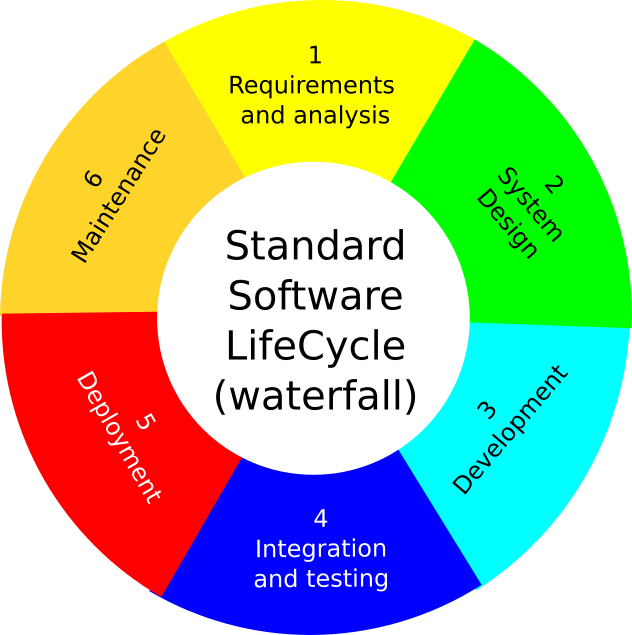
Requirements and Analysis: Requirements are gathered from stakeholders (client, users) and analysed. From this, the Software Requirement Specification document is produced outlining all functional and non-functional requirements. The focus here is on understanding what the system is supposed to do without going into the details of implementation.
System design: The blueprint for building the system is created. It identifies the architecture, the components and their interaction but also focusing on the details of each components (data structure, databases, input/output).
Development: This is where the coding takes place. The developer will write the code based on the Software Requirement Specification to ensure that it meets requirements. It is often done iteratively by creating small building blocks.
Integration and testing: Once the code is written, the individual block are integrated to a complete system and various testing are conducted (e.g. unit tests, integration tests).
Deployment: Once the software is fully tested and ready, it is deployed to the production environment or delivered to the client. It is at this stage that the software is available to end-users.
Maintenance: At this stage, the software enters the maintenance phase where it is monitored, updated and fixed if necessary. Corrective maintenance fixes bugs, while adaptive and perfective maintenance improve the software’s performance or add new functionalities.
It is quite a rigid framework that does not really adapt well to changes. This framework is often called a waterfall model. Nevertheless it is possible to adapt it to research the academic framework. That what we will see in the software management plan in the next chapter.
Content from Software Managagement plan: Planning ahead
Last updated on 2024-11-08 | Edit this page
Estimated time: 10 minutes
Overview
Questions
- What should you ask yourself before starting a software project?
- How do I write a Software Management Plan?
- How do I disseminate my work and make it findable?
Objectives
- Understand the main points of a software management plan.
- Know the main questions you should ask yourself before diving into coding.
Introduction
A Software Management Plan (SMP) is a framework that outlines how software development, releases, maintenance, and support will be conducted throughout its lifecycle. It outlines how the key aspects like goals, resources, milestones, risks, and quality are effectively controlled. The plan is usually created early in the project, ideally before work is undertaken, and is essential for both small and large projects to ensure clear communication and expectations among team members.
This chapter presents various point that you should think about when you start developing code for research. The goal is that you ask yourself the right questions before embarking on your software development journey.
Software Management Plan
Research is quite different from the private sector. The goal of a research software is to answer a research question in a way that allows other people (and yourself) to carry it forward. Aligning the SMP to the research lifecycle ensures that the software not only facilitates scientific progress but also meets academics standards for collaboration, documentation and reproducibility.
Project Overview
You will not start a research software project without having a research question you want to answer. Instead of business requirement that you would have in a traditional software lifecycle you should therefore gather research objectives. Having a clear research question will greatly help you define the requirements for your software.
But how do you proceed? The answer is the same as for the research question: you look at what is already out there in the literature and software ecosystem of your domain. If someone has already answered your research question then you typically would not try to answer it again (unless seeking to replicate the finding). The same is true for research software: you will first look at what is already available. The case where people are re-implementing something that already exist is far too common and wastes a lot of previous research time and funding. In short: DO NOT RE-INVENT THE WHEEL. If something is already available but does not have all the functionalities you are looking for then maybe you can build upon that previous work. If the code is open source, you might just be able to clone it and carry it forward (as long as you give credit to the original author). Alternatively you may be able to contribute features up-stream to the package you wish to use at the same time forging connections and potential collaborations. If it is not open source, you might be able to contact the author and ask if you can have access to the source code, again this may lead to fruitful collaborations!
If you still want to create something from scratch when something already exists, always ask yourself: what would make people use my solution instead of the one that is already available? What benefits will my software bring to the research community?
When you have a good sense of what you want to create you must define what data will be used as input and what the output of the software will look like. It is common that for a given domain some data standard are already in use. Try to stick to these standards as much as you can as doing so will make your software more Interoperable with other systems and Reusable by others.
Human and Technical resources
Once you have a good idea of what you want to create, the next step is to identify who is in charge of it. Identify team members (e.g. P.I, Research Software Engineers (RSE), Postdocs, PhD students) and define their roles in the development of the tool. It is often useful to extend the distribution of roles to other aspects of the project, such as data management & publication (it is important that everybody gets credit for their work and can evidence it). Doing this before you start you will help you identify what skills are available in your team and what, if any, deficiencies there are and hence identifying the needs for training. Discussing these aspects beforehand will save you time and potentially help avoid some painful discussions (and frustrations) down the line.
One crucial aspect of a software project, especially occurring in research, is the problem of the bus factor. Simply put it is a measurement of risk that arises if a member of the team were “hit by a bus” and the information and capabilities they hold about the project has not been shared with the team. The worst bus factor is 1 and means a single person is in charge of everything. If you lose that person then the project stops as no one else knows about the project or has the skills to continue working on it. The higher the bus number the better as it means there is shared knowledge and skills within the team. However, trying to mitigate this bus factor is crucial, and sometimes very hard to do, particularly in smaller research groups. There are some steps that can be taken though, for example you might want to have different people within the team familiar with the code. This can be achieved through regular peer review during the development cycle. Perhaps most important is to have very clear and up-to-date documentation on what the software does and how it is used. The module on Documentation will be helpful in how to go about this.
On the technical side, it is important to identify technical resources, e.g. computing environment, High Performance Computing platform, that might be available. You might not see their need at first, but as development proceeds if you know what support you can get from your own institution it will be useful know where and how accessible the support is as some, such as Research Software Engineer support, may need financing and you would need to plan this in advance as part of the budget.
Development Approach
How will you develop your code? Multiple ways exist to manage the development of a software, we can name two here:
Waterfall: This approach is a linear and sequential project management technique where each phase depends on the completion of the previous one. It consists of stages like requirements gathering, design, implementation, testing, deployment, and maintenance. Progress flows in one direction, similar to a waterfall. Changes are difficult to implement once a phase is completed. It is best suited for projects with well-defined requirements and minimal expected changes.
Agile: This approach is an iterative and flexible project management technique focused on delivering small, incremental improvements. It encourages collaboration between cross-functional teams, frequent feedback, and continuous adaptation to changes. Work is divided into short cycles called sprints, with regular reviews to assess progress. Agile prioritizes customer satisfaction through early and continuous delivery. It is best suited for dynamic projects with evolving requirements.
Whatever method of project management you choose, ensure that it is fostering collaboration within the group. Both have their advantages but keep in mind that research is an iterative provess involving a lot of trial-and-error cycles and experimentation. Communication will be a key aspect of success. It might be interesting to have short development cycles with an iterative approach in which case an Agile approach would be more suited. This will help you analyse intermediary research outcomes, review the development of the software (and documentation) and overall progress and regularly review and realign the goals if necessary. It is not rare that while developing a research tool with a clear goal in mind you end up finding serendipitous results that are worthy of publication in their own right!
The tools are you use for software development are also very important. Do not end up with multiple files for the multiple version of your software (e.g. version1, version 1_1, version1_2, version1_final, version1_final2, etc.). It will become unmanageable. To avoid this situation, it is important to have a version control tool that allows you to track the changes that you implement in your software over time. Using version control will help making your software more FAIR. If you are unfamiliar with version control then the modules on Git for Beginners and Git Collaboration will be useful.
Timeline and Milestones
Creating software is not easy. The bigger it gets, the more complicated it becomes. Hence it is important to have checkpoints, or milestones, that you can work toward. It is often possible to split the development of a software package into multiple intermediary states. These intermediary states will give you more realistic and achievable objectives and allow you to track progress and allow you to create your development timeline.
It is important to coordinate this timeline with research milestones and deadlines. They can be paper submissions, conference presentations, funding deadlines, PhD thesis submissions. Software development and research progress should be as aligned as possible and tracked concurrently. If they diverge you will be in trouble.
Risk Management
Spoiler alert: nothing will work from the first try! You will inevitably encounter blockers which hinder progress during the development of your software and only some of these will be identifiable beforehand. Failure to achieve scientific outcomes, data issues (sometimes data will never arrive!), reproducibility failures, or tool/library obsolescence are examples of risks that can be anticipated.
Once identified, you should think about how to mitigate these risks and create a contingency plan (a plan B) in case they turn out to be real problems (e.g. backup strategies for data and code, alternative methods).
One important aspect of research it is that it is hard to plan. So how you address changes in the research hypothesis, data, or experimental design and how this might impact software development is important to consider. Ensure proper version control for both code and datasets and allow for flexibility for exploratory research where new insights might lead to changes in the software. This gets much easier when the code is written in a maintainable, readable and Reusable way. If unfamiliar with software development the module on Design your code will be useful.
Quality Assurance
Wouldn’t it be sad if all the work you have been putting in your software development is wasted by a typo in the code? How do you make sure that the code you are writing is functioning as intended and that the scientific results your producing are reliable and reproducible?
There are some strategies to ensure both code quality and research reproducibility:
Peer Review : Code quality can be checked by doing code peer reviews. Regular peer review are very helpful both for increasing the quality and for disseminating knowledge in the team (reducing the bus factor). Automated testing with continuous integration. There are modules on testing and continuous integration and version control (Git for Beginners and Git Collaboration) that can be undertaken.
Data Version Control : Reproducibility will benefit from the previous suggestions, but you can also adopt a strategy for data version control (DVC) that will help track the state of your data and changes. Parametrisation of code ensures that it can be rerun with different parameters easily (without changing the code) via configuration files or command line interface. The module on Code Design covers how to do this.
User Testing : Testing by other people also helps. If someone else in your team can reproduce your results it will give you a good idea of how reproducible are your results. For a more advanced case, it is possible to fully automatise reproducibility checks and strategies for this are covered in the testing and continuous integration module.
Reliability : The reliability of scientific output can be tested by comparing your code with existing datasets. Can you replicate existing results with your own tool? That will give you an interesting insight.
Tested software is more reliable and robust and thorough testing of your code will greatly enhance its Re-usability and make your science more Reproducible.
Dissemination
Dissemination is a cornerstone of research. When it comes to research software you will have to make sure that people know where to find it and how to use it (Findable and Reusable). It comes down to three important pillars:
License: This is probably one of the most basic items of the software management plan. If there is no license for your software people won’t know if they have the right to reuse your software. No license means that you retain all the rights to your source code and no one may reproduce, distribute or create derivative works from your code (which is the opposite of research philosophy). Please see the chapter on intellectual property and software licenses for more details [link TBD].
Documentation is paramount, the main group that will help is your future self and members of your team! Research projects often span several years during which new members will join and some will leave the team. If it takes someone 6 months just to understand the software that the team is developing that is wasted time. If you want a sustainable project, write good documentation. In addition, If you produce software with a good documentation it will be more likely used by the community and then you might get credit for it! Make sure that the documentation is easily accessible (e.g. a website) and tailored to future researchers and the academic community in your research domain. You should describe the research question the software seeks to solve as well as how the software works and should be used. The module on Documentation covers advice on how to document software projects.
Software Repositories: It is important to place your software (the released version) to a place where people can download it freely. Collaborative development platform such as Github and GitLab are not made to store software on the long term. More persistent platforms designed for the sharing of research output exist such as Figshare and Zenodo and should be used for long term storage of software. Please see chapter on dissemination for more details [Link TBD].
To go a little bit further, you might want to take extra step in the dissemination direction:
Publication: Once you release your software, you might want to publish it! Numerous journals accept papera about research software. Some are generalists (e.g. JOSS, JORS, SoftwareX to name a few) and some are domain oriented (you can get a non-exhaustive list from the Software Sustainability Institute)). A dedicated paper describing your software is really something you should consider! It will give you a lot of visibility and allow you to get a citable paper associated to your software (you can follow the lecture on [Publishing your software] for this).
Training: If your software sees a lot of buy-in from the community and is relatively complex to use (not all software is), you might want to train others to use it. You can do that via tutorials and/or training events. This is a very powerful way to give your work more visibility and a very efficient way to create collaborations!
Long term sustainability
Maintenance of the software is tricky. There are two possible scenarios:
The software is in a final form, but the funding is still there: In that case it is important to identify who is in charge of the maintenance and update. You might be working with totally different people than at the beginning of the project. So it is important to plan ahead for this in the Software Management Plan.
The research project comes to an end (and with it, its founding). That’s the tricky situation, long term maintenance is difficult because funders do not plan for this (which happens everywhere). If you want to increase the chances that your software works in 5 or 10 years, what you could envisage to do is to distribute your software with its own computational environment. This ships your software with the required dependencies and put all this in its own bubble. This greatly enhances the long term Reproducibility of your work. If you are interested to learn more about this, you can enrol in the Reproducible Computational Environment.
In either scenario having accessible and detailed documentation will be really useful for the long term maintenance of the code.
Minimal version
Everything that has been described previously can be quite intimidating at first. There is a lot to think about and depending on the size of your project you might not need all of these details. If you want to go with a minimal set of items to put in place you could use the following:
- Information on what software outputs (including documentation and other related material) are expected to be produced.
- Who is responsible for the development work?;
- Which version control system is to be used?
- What license will be used for each produced piece of code/data?
These four items should be on your list in the planning phase.
Content from Copyright and Licenses
Last updated on 2024-11-08 | Edit this page
Estimated time: 10 minutes
Overview
Questions
- What is copyright and intellectual property?
- What license should I use for my software?
- How do I licences a work that has dependencies?
Objectives
- Understand the legal terminology around intellectual property
- Understand the main software licences, their differences and how to choose one
- Understand how to work with licences and dependencies
Available soon.
Content from DOIs and Repositories
Last updated on 2024-10-11 | Edit this page
Estimated time: 30 minutes
Overview
Questions
- What is a DOI?
- What good is a DOI (compared to, say, a URL), and how do I get a DOI?
- What is a repository?
- How do I choose a repository?
- How do I link GitHub to ORDA?
Objectives
- Understand what a DOI is
- Understand how they differ from other ways to link to your work and how to create and source a DOI for your work
- Understand what a repository is and does
- Feel comfortable selecting a suitable repository
- Know how to link a GitHub repository to ORDA (a DOI-providing repository)
DOIs
Introduction
The code that you write is important. No matter the size, style, or language, it is an integral aspect of the research that it is part of, or indeed, sometimes it is the main output of the research project.
Historically ‘research outputs’ have been focused on papers, and while this is still mostly true, there has been a change in recent years to give better acknowledgement to other research outputs, such as code. There has also been a shift (which we are continuing to see) that funders are requiring other outputs from projects to be made available. While this is mostly focused on the data that has been collected or used, in certain fields code is also part of this. You may also come across conferences or awards that require code to be available (and open) in order to submit or be considered. There’s also been a rich history of code being made free and available to others.
Regardless of the reason you want to disseminate your code, there are some ways you can make this easier for both yourself and anyone who wishes to use your code - these include DOIs, using repositories, providing metadata, and knowing how to cite yourself.
What is a DOI?
A DOI is a type of PID. A PID is a Persistent Identifier - a reference that will continue to identify the same object, person or institution over time. A DOI is a Digital Object Identifier - a PID that refers specifically to a digital object such as an online article, dataset or archived piece of software. Each individual DOI is linked to a specific digital object and (should) always direct to that object, regardless of potential changes to its location or metadata. The most common use of DOIs is for journal articles. A single article should have a single DOI, but they can also be used for databases or software/code.
Example DOI
This is what a DOI looks like: 10.1016/j.ascom.2020.100427
You’ve probably seen these many times before because, like this one, they are used for journal articles. Interestingly, the article the above DOI links to is about a tool that has been created, is freely available, and has a licence applied to it, but it provides no link to the code/software itself.
Isn’t that just a link/URL?
No. The internet as a whole is much more fragile than most people think. There area myriad of different ways that things can break or cease to exist. While there are, of course, some old webpages that are still up for no apparent reason, and multiple archives and projects that try to capture parts of the internet (Wayback Machine, Software Heritage), these are by no means complete.
But thinking of your own code, if it’s on a webpage (maybe your own), hosting isn’t free: are you planning on keeping this webpage for a long time? Can you guarantee it will never be moved from that page/URL, and do you know if other people are using that link?
Callout
A DOI essentially makes it someone else’s job to ensure that the link resolves to the right digital object.
Only registered organisations can create DOI’s. To be able to mint a DOI, an organisation must meet the International DOI Foundations standards and pay to become a member, meaning that they must have the ability to ensure the DOIs are maintained.
DOIs are also designed to be short and human-readable, again unlike most URLs, whereas shortened URLs are not persistent.
DOI and version control
Version control is of course very important around code (see the Version Control part of the FAIR24RS training), and you shouldn’t create more than one DOI for a single digital object - however you can nevertheless version with DOIs:
DOIs with multiple versions
That part at the end indicates which version the DOI will resolve to. If you have written some code for a research project, and the current version is the one that was used, but you are still developing it, referencing that version in any paper or outputs will allow people to see exactly what the code looked like at the time the research was conducted.
It’s important to note that if no version suffix is included in the DOI link, it will resolve to the most recent version of the object. This is sometimes exactly what is wanted and sometimes not, so it’s good to think about this when using a DOI.
How do I get a DOI?
To get a DOI for code, the best place to use is a repository. It’s important to note that (currently) GitHub does not mint DOIs. Some examples of repositories that do are Zenodo and the University’s institutional repository, ORDA.
When you make a deposit in a repository that does mint DOIs, there should be nothing extra you have to do, when it has been published, there will be a DOI that you can use to cite and refer to your work.
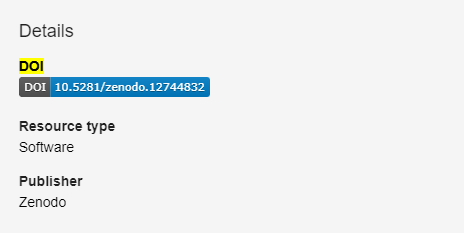
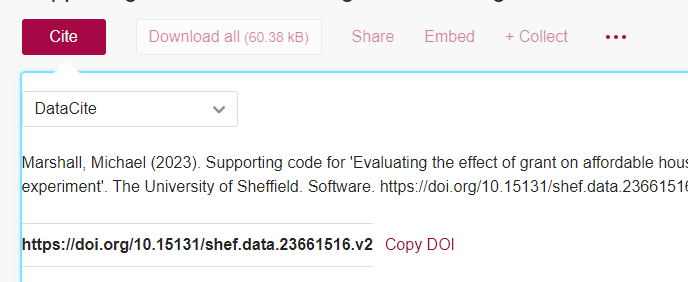
Key Points
- DOIs are persistent identifiers that should always point to your code.
- They should be maintained by the minting organisation, making them stronger than a URL.
- DOIs can handle multiple versions of the same object.
- Some repositories mint DOIs for deposits.
Repositories
Introduction
Hosting large amounts of data, minting DOIs, creating a usable interface for code and the ability to pull the code to other work setups all takes a lot of time and effort. This is where repositories come in. A repository will make space available to you to deposit your work. GitHub, as a whole, could be seen as a repository, although you also create repositories (repos) yourself inside GitHub. It also does a lot more than just hold your data/code, and of course its main function is version control.
In the last episode we saw some repositories - Zenodo and the University’s institutional repository ORDA - but there are many more out there. Most repositories, certainly used in academia, tend to be fully open, so anyone can access what is deposited.
It’s important to remember this, as you may not want, or be able to, make your code fully open to all (although we encourage you to where possible). However, there are repositories out there that have different levels of access. For example, the UK Data Service repository has three levels of access: open, safeguarded (users must sign the End User Licence), and controlled (users must apply for access with criteria set by the data provider). However, currently the UK Data Service does not hold code.
It’s also worth noting that there are different types of repositories. Some are purely for publications (such as the White Rose Research Online repository), whereas others are data-focused (e.g. Harvard Dataverse).
Differences between repositories
As we’ve just seen, not all repositories are created equal. Some are not just repositories, others aren’t technically repositories at all. This means you should put some thought into which repository you are going to use to deposit your code.
What do you want from a repository
- Do you want your work to be fully open?
- What type of licence do you wish to apply to your work?
- Do you want a DOI?
- Do you need it to handle versioning?
- Do you need your work to be machine-accessible?
You may also need to think about what licence you can apply, as you may be limited by any third party software you are using. This is covered in more detail in the Software Licensing section of this component.
Also the last point is probably more important around data rather than code.
Choosing a repository
As there are many different repositories out there, choosing a suitable one for your code is an important task. You may know the repository that you wish to use already, or you may wish to find a suitable subject-specific repository. Both re3data and FAIRsharing hold a directory of repositories that can be browsed, searched, and filtered to help you find the most suitable repository for your needs.
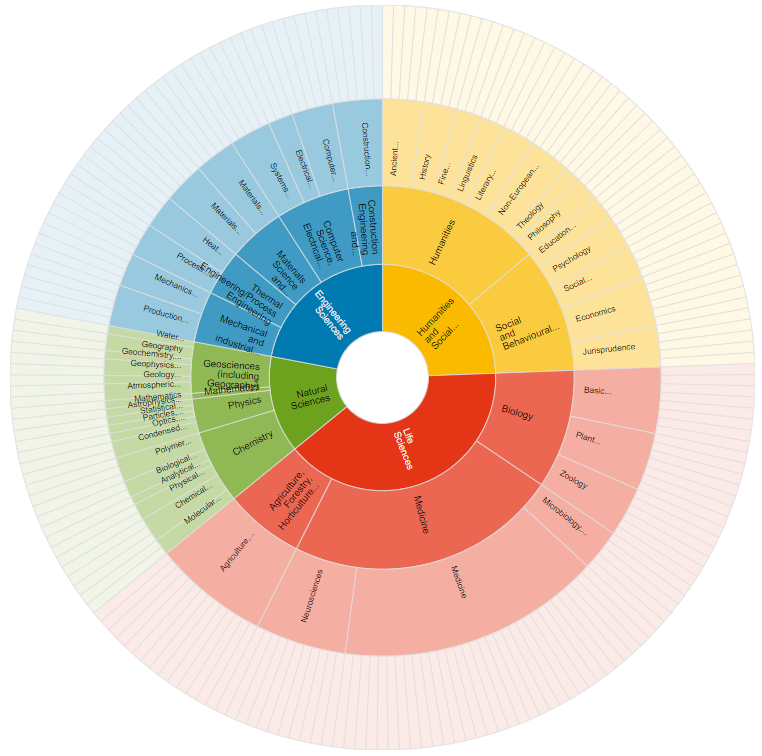
Both have a useful description of each repository and helpful icons to enable you to quickly answer some of the questions listed above.
Our own guidance is that you should look for a subject- or data-type-specific repository first, and if nothing is suitable or has the functionality required, then you should use ORDA (the University of Sheffield institutional repository).
Challenge 1: Would making my code a package be better?
If one of the main aims is to make the code you have written more (re-)usable by others, would it not be more suitable to create a package of the work, and what would be the pros and cons of doing so?
Think about who ‘owns’ packages, how they are disseminated, and what we wanted to achieve with our code
Maybe! But also probably not. The main issue is that they are different things really, or at least help and aid in different ways, but use a lot of the same vocabulary. While a package will most likely make it much easier for people to use you work (a simple install command), it often doesn’t make it more citeable.
For example, the main repository of Python packages - the Python Package Index or PyPI - holds over half a million projects, but does not mint a DOI for these projects. However, recently, all R packages on CRAN have been attributed a DOI. Now of course there’s differences there in how packages are regulated, but it’s a good step forward in giving the creators of packages the recognition they deserve.
This is also the issue with using just GitHub that while it holds all your work, and enables people to access, read, use, and reuse your work, it also does not mint DOIs, as its main function is to aid in version control and allow a team to work on the same project at once. Therefore, unlike other repositories, it is not so concerned with the long-term storage or archiving of work , and, while nothing has happened yet, it’s also worth remembering that GitHub is owned by Microsoft and could technically change at any time.
You can learn a lot more about packages in the FAIR24RS component on this topic, including how to create and publish your own and how to automate the publication.
You may also consider the fact that while usually it is good practice to only put your work in one repository, it might be beneficial in this instance to have a package created for easy download, and also place your work in a repository that grants a DOI for better citation. (Note: it’s bad practice to gain more than one DOI for an output).
Can this not all be automated and made simple?
There are certainly things you can do to help you manage this, but it depends on your definition of simple.
Within ORDA, you can link your account and your GitHub account.
Challenge 2: Go to ORDA and find the button that imports from GitHub
See if you can find the button for linking a GitHub repo in ORDA
We’ll go through this in a minute, but if you did actually find it, well done! Figshare (of which ORDA is an instance) does a lot right, but has some very strange decisions inside it as well.
ORDA
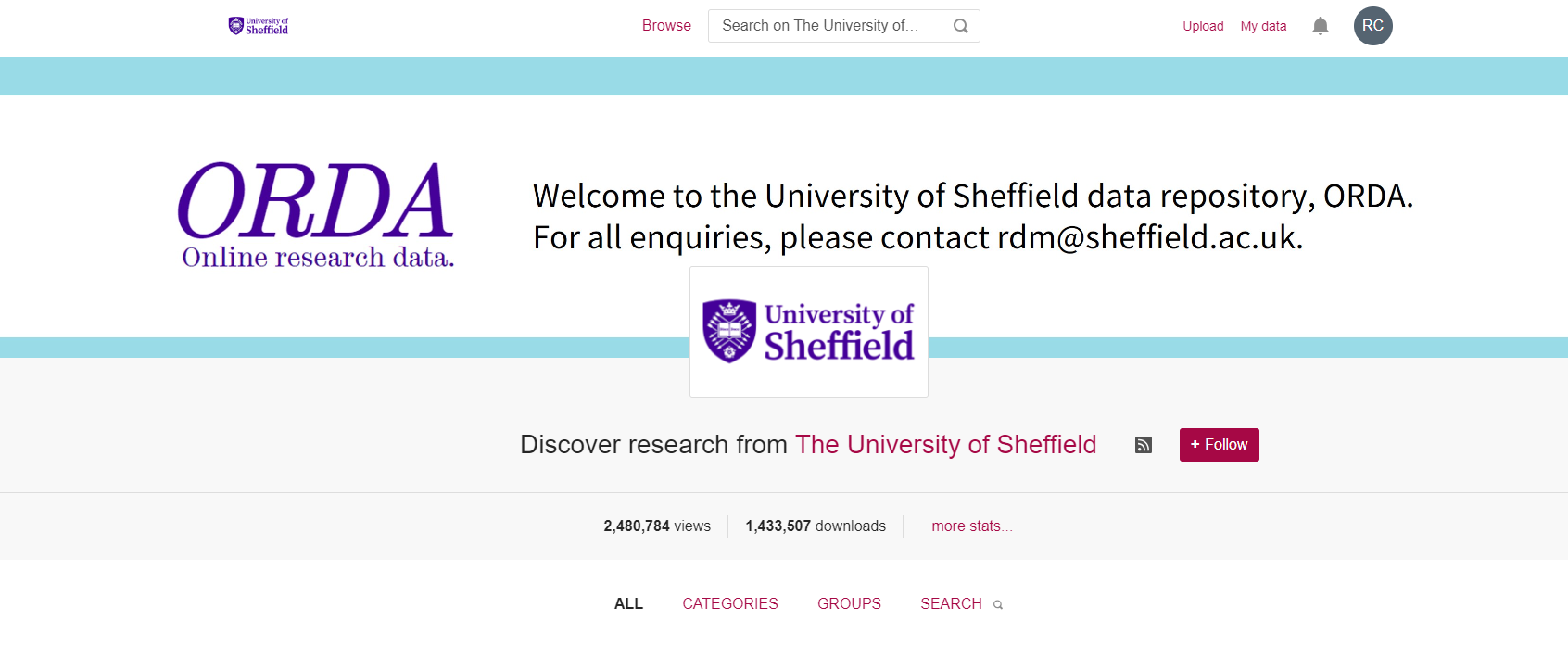
This is the ORDA landing page, showing my login. All University of Sheffield staff and PGRs should have an account to access ORDA as it is associated with your staff/student profile (N.B. it is also deactivated with your profile if you leave, but can be reactivated for a period if needed to allow a deposit or edit to be made).
From here, if you go to the My Data section in the top right, you will see something like this:
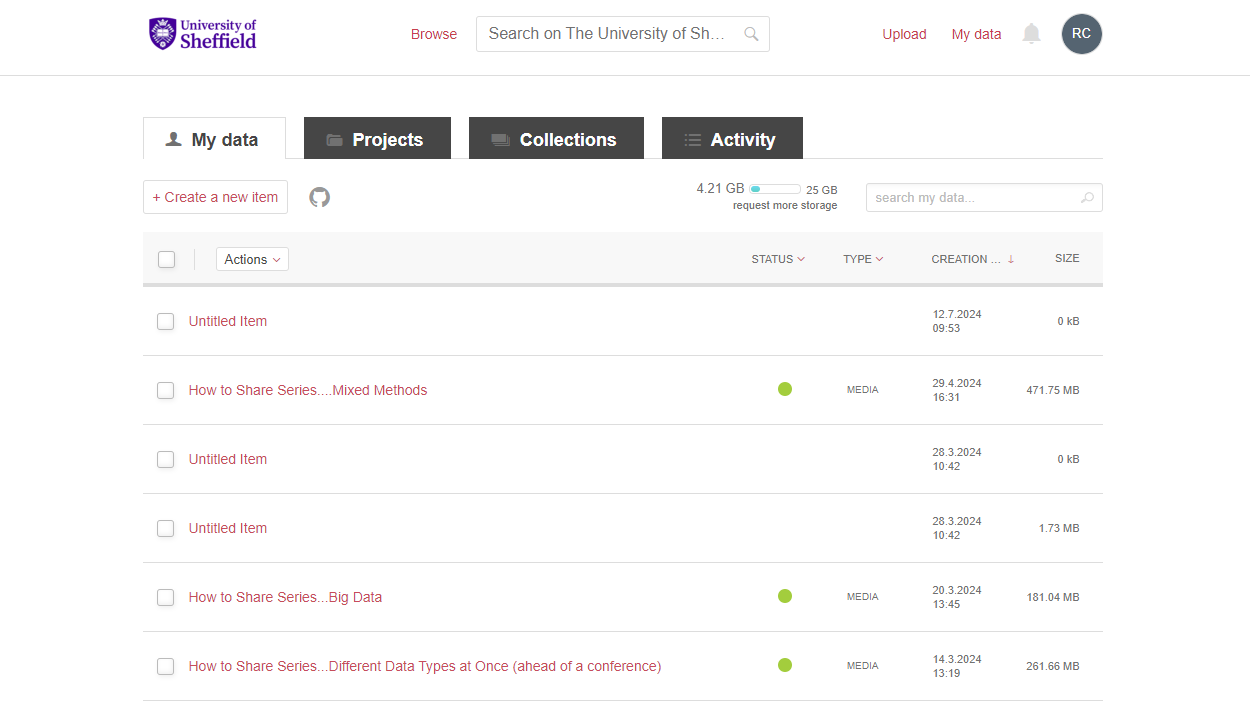
You might be able to see it now, but it’s still not clear how useful that button is. Next to the ‘+ Create a new item’ button is a greyed out Octocat. Hovering over this will fill in the colour and provide the helpful words ‘Import from GitHub’. Clicking on the icon will give you this popup:
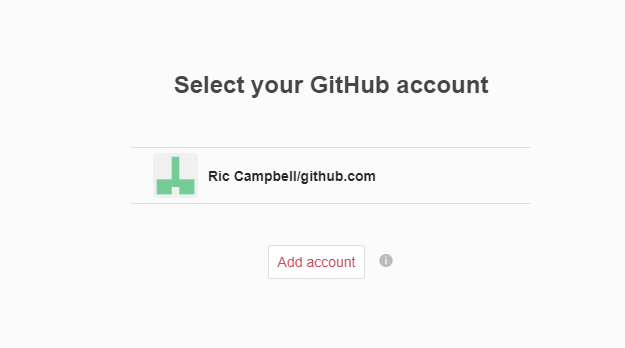
And from this you can link your account, and pull in a repo directly to ORDA. You can see from the image below that it simply allows you to select which repo you want to pull across.
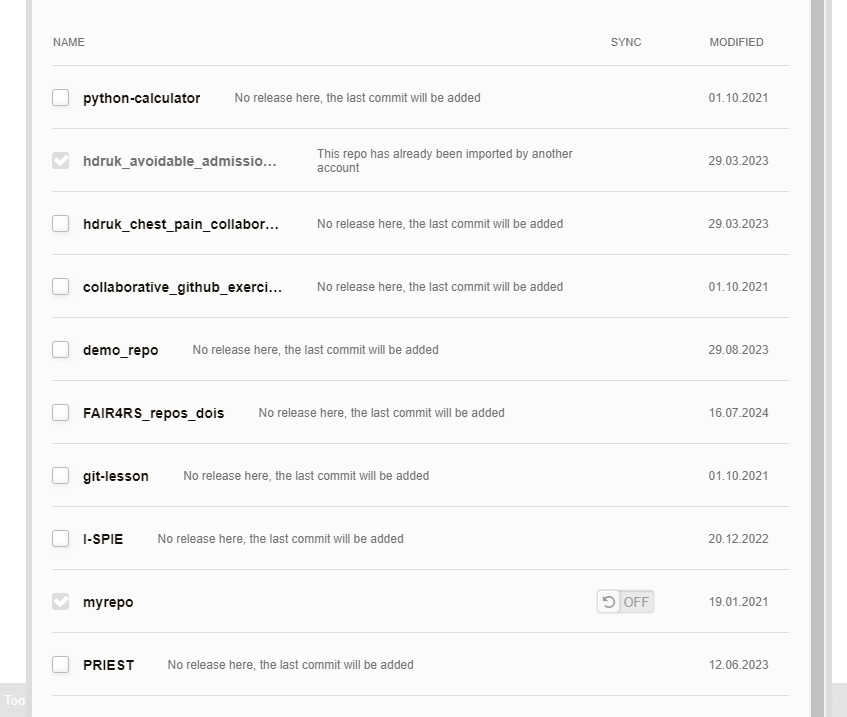
There are a few other bits of interest in this image as well. First, you can see that if a GitHub repo has been imported by another user, it doesn’t let you create another deposit for it (remember, this would mint another DOI for the same output), and this is across all instances of Figshare (for example that one is in the main Figshare.com instance). There is also a simple option that you can see there that allows automated updates of your GitHub repositories to be pulled into ORDA: the slider between the repo name and last modified date.
However, that automation is reliant on the use of a GitHub Action, which might not be the best approach for you (guidance on the Action).
Alternatively you could use Figshare’s API to automate the creation of a new version. There’s a much more detailed description and example of how to do this in the Packages component of this course.
As mentioned above, if you are thinking of having both a package in a repository, and depositing somewhere else to get a DOI, this is something you give good consideration to, as they may be extra work to ensure that there are not different versions of your work in different places.
Zenodo
Of course, these are not the only places you can use. Similarly in practice, you can deposit a GitHub repo in Zenodo. GitHub provides guidance on how to do this.
Key Points
- There are numerous repositories available and you should consider what you want from one
- Some are better than others depending on your purpose and needs
- GitHub doesn’t mint DOIs, but you can link your GitHub repos to a repository that does
Content from Metadata and Citation
Last updated on 2024-10-11 | Edit this page
Estimated time: 15 minutes
Overview
Questions
- What is metadata and why is it important, and what is best practice around metadata?
- What does good metadata look like?
- I can cite/people can cite my code?
- What do you want people to access if it’s cited?
- How do I cite code?
Objectives
- Understand the role and need for metadata for your outputs
- Know what best practice is and be able to apply it
- Learn from examples of good metadata
- Understand the importance of citing all work
- Be able to think about what should be cited
- Know how to cite code, and how others can cite you
Metadata
Introduction
Simply put, metadata is data about data (hence meta). This might not be that helpful a description however, but is necessary as it holds true across all types of metadata. If we continue with the idea of depositing your code in a repository as we saw in the previous episode, however, it becomes much clearer. The metadata is the data that’s on the deposit ‘front’ page, so to speak. It is there to inform anyone who comes across the deposit what they have found without them having to dive too far into the actual data or code.
Can you show me some good metadata?
Of course, however, it might be more interesting to look at some bad metadata, as it’s probably more effective in showing how important metadata can be.
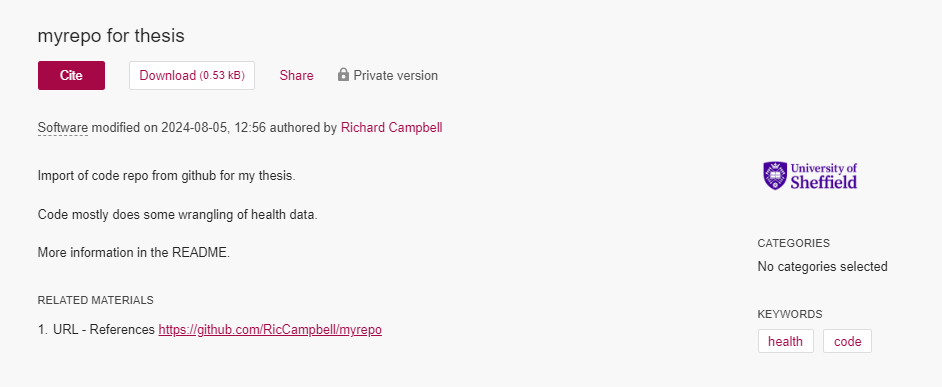
As you can see there, there’s some information about the deposit, but it’s probably not nearly enough for anyone to know what it really is, or if it’s useful to them. This code could be perfect for someone to use, or adapt, but they wouldn’t know without having to do a lot of work to figure this out. There is a link to the GitHub repository, but that’s probably not got any more information in it, and still, as we’ve seen, that wouldn’t enable someone to reference your work. (N.B. There’s no DOI on this deposit, as it’s just a draft - we wouldn’t really accept a deposit into ORDA that had so little information - another good reason to think about metadata, as ORDA isn’t an outlier in this regard).
But there is a README there, which asks the question:
Is a README metadata?
It’s a good question, and one I’m sure people would argue over as they have different views on it. Technically, metadata is for finding software, while documentation, including READMEs, are for understanding it. However, the thing that actually matters is that you should probably have a README, and metadata, and other documentation as well. READMEs and other documentation (comments etc.) should be created early and updated as you go along, with potentially metadata being created later in the lifecycle. However, a good README may directly feed into your metadata. There’s no problem with copying and pasting elements; in fact it can be beneficial: it saves you time, and makes sure everything is consistent.
It might be worth thinking of metadata as the overarching information about your code. A README goes into more detail but covers the whole software/project, and comments etc are much more fine-grained documentation relating to the places in the code where they reside.
There is a Documentation module on this FAIR24RS course, which goes over all things documentation in more detail.
Challenge
What metadata do you think should be included in a deposit?
Think about what you might want to see if you were reusing code (i.e. a package), or what you probably take for granted that you know about your code
- Name (a good one)
- (Lay) summary
- A description of what it is
- What language is it in, and versions
- Provenance
- It’s licence (see licence section)
- Any related material
- Dependencies
- File structure
These might not even be everything depending on what you have created. You might want to have use cases, the target audience, key features, compatibility etc. The point is to have information that reduces the workload or thought that someone has to take on in order to know what it is they’re looking at, and if it’s useful to them.
None of these are world-changing ideas, but they do require some thought:
Callout
“There are only two hard things in Computer Science: cache invalidation and naming things.”
– Phil Karlton
You may have heard or seen this quote before, but it does stand true: naming things is hard. This principle is normally applied to variables, but it does carry over to other elements too. Creating a short, informative summary or description can be tricky, but doing that using no specialised vocabulary makes it harder, as does trying to include all the less tangible, or overarching, elements that you know about your work but others do not.
If we go back to the example of bad metadata that we saw earlier, there are actually two bits of information there that are helpful.
- It’s got the right type of item type selected, as it’s down as ‘software’, which makes it easier for people to find and know immediately what it is.
- It also mentions that it’s mostly to do with ‘wrangling of health data’. This is not much information, but it can be very easy to forget the most simple elements. If you’ve only ever worked with health data and written plenty of bits of code to help, you might not think to mention this very high-level piece of information.
Machine-findable
It’s best when thinking about creating metadata that you think of human users. It’s also really good to think of yourself as one of these users, either yourself a few years ago, when you might have known less of the area and might not have known exactly what you were looking for, or yourself in a few years, when you’ve most likely forgotten everything about this code, why you made it, and what exactly it does.
However, you also get a bit more findability for free by creating metadata, as these fields are often used and standardised for machine readability as well.
A good example
We’ve seen a bad example, but what would a good example look like?
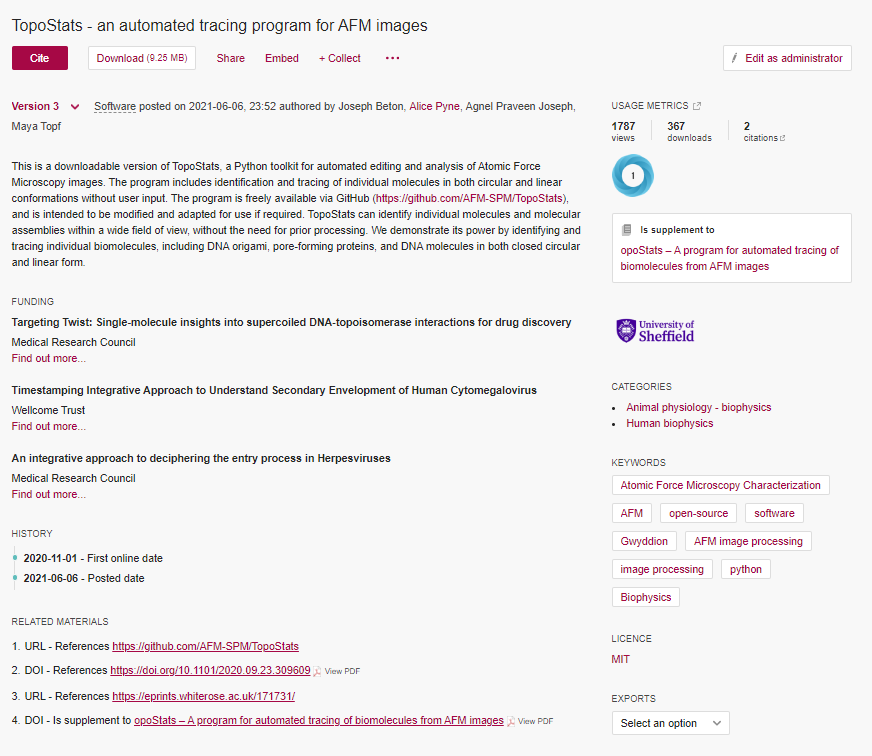
Can you pick out the good (and bad) elements of metadata in the above deposit?
- Author names
- Version number
- Date created
- Item type
- A clear name
- A clear description
- Licence
- Categories and keywords
- Related materials including a link to the relevant paper
- Initialism in title (bad)
There’s also the language that it is written in, both in the description and the keywords. The first three listed, names, version, and date, would all fall under what was previously referred to as provenance. As you can see, a lot of these are fields you probably have to complete when making a deposit to a repository, which makes it easier, but if you are taking a different route, these could be easy to miss out. It’s also important that you keep these items up to date if they change. Do also take a bit of time to consider them - for example, it’s not good form to miss someone off an author list, and this applies to software author lists as well.
Standards
As we’ve just seen, a repository, even a GitHub repository, has fields for some of this information and these will be implementing a standard, but if you are adding metadata that isn’t a standard GitHub/repository field, or creating your own metadata document, you might need to consider using a standard for your metadata. Some examples are schema.org’s SoftwareApplication type, and CodeMeta. These help tools to work with your metadata by having a set standard. For example if you created it now, what would you call your author list field? Possibilities might be author_list, authorList, developer, developers, writer, creator, coders etc., are all these synonymous with each other for everyone? Would you complete those fields the same for your project? Implementing a standard stops this from happening, each field has a precise meaning and information on how to complete/read.
You’ll have come across standards before, of course, when you download citations of papers for your reference manager software:
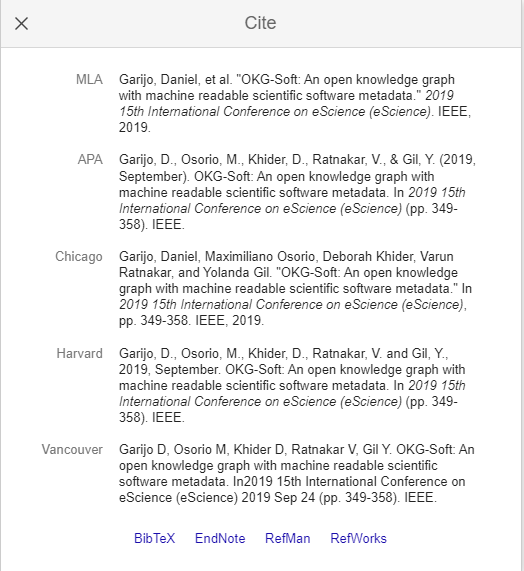
Key Points
- Metadata helps drive discovery of your software
- It’s easy to not include key elements as these are ‘obvious’
- Standardisation of metadata is useful to help tools use the metadata
Citation
Introduction
If we go back to the first thing that was stated in this module,
The code that you write is important. No matter the size, style, or language, it is an integral aspect of the research that it is part of, or indeed, sometimes it is the main output of the research project.,
This also applies to code that other people write. That’s why it’s important to know that you can (and should) cite other people’s code when you use it, and also how to enable other people to cite yours.
DORA, funders, and the (hidden) REF
DORA, The Declaration on Research Assessment, sets out to improve the ways in which the outputs of scholarly research are evaluated, essentially moving away from a focus on the impact factor of journals that you publish in. The University of Sheffield is a signatory of this declaration. DORA prompts institutions and the sector as a whole to consider different ways of evaluating research quality, including assessing the quality of all research outputs (e.g. datasets, code) as well as published journal articles, and encouraging recognition of all authors’ contributions.
We’re also seeing a continued movement from funders to make open and available all aspects of research projects as the importance and cost of all elements are more fully recognised. For instance, we’ve seen this with data, with a lot of funders requiring (where suitable) this to be made openly accessible, and we’re also increasingly seeing it with code. Making things open and reusing existing work are both important goals, and ensuring people get the recognition they deserve from their work is essential (and also in most cases a legal necessity - see licence section of this module, or software Terms and Conditions e.g. SAS).
There’s also been some movement with the REF as well, with more types of outputs being considered eligible to be returned, and if we think about impact case studies, a useful piece of software could easily have a substantial impact. There are also movements like the Hidden REF, whose objective is to gain recognition for all people who contribute to research, and are also wanting institutions to pledge to making 5% of their REF returns non-traditional research outputs (i.e. anything that’s not a journal paper or book).
These are also all good reasons not to simply cite the associated paper for a piece of software (if there even is one), but to cite the software itself - and to make it easy for others to cite your own code.
Citation and CITATION.cff files
Citation of software is of course different to that of a standard journal article. Normally there is one version of an article and nothing that can really ‘break’ with them; this is not true of software. Also, a paper tends to have all the information you need to cite it correctly quite clearly there for you - again, this often isn’t the case for software.
Challenge
Think what people might need to know from a citation to be able to reproduce a piece of research. (I.e., to make sure they’re using the same piece of software used in the original study?)
Some of the main things to know about the software used that would be integral to being able to reproduce the outputs would be:
- Title
- Authors
- Version used
- Where to find the software
- How to access the code (if different to the above, and available)
The first two, while it’s clear that they should be part of a citation, might not immediately seem relevant when thinking about reproducibility, but if you think that they can sometimes act as a kind of ‘primary key’ to help people know they’re working with the right software (see the last episode and its discussion of naming things).
The bottom three you can see would be needed in order to be certain you can reproduce the work as best as possible. We know that many changes can happen between versions, some big changes, some small changes that could have knock-on effects to how everything works. Of course, where to find the software goes back to our first episode, and having a solid pointer to the code can be solved by getting a DOI for your work (you should of course not mint a DOI for other people’s work, and you might just have to cite the best link available).
CITATION.cff
files are designed to pull together
everything you or others need in one place. Similar to README, or
LICENCE files, they are top level files that contain in a standardised
fashion all the information required.
This is an example of a CITATION.cff
file (the one for
this module):
This CITATION.cff file was generated with cffinit.
Visit https://bit.ly/cffinit to generate yours today!
cff-version: 1.2.0
title: 'Software dissemination and impact'
message: >-
If you use this software, please cite it using the
metadata from this file.
type: software
authors:
- given-names: 'Jenni'
family-names: 'Adams'
email: 'j.adams@sheffield.ac.uk'
affiliation: 'University of Sheffield'
orcid: 'https://orcid.org/0000-0003-2420-0763'
- given-names: 'Ric'
family-names: 'Campbell'
email: 'r.j.campbell@sheffield.ac.uk'
affiliation: 'University of Sheffield'
orcid: 'https://orcid.org/0000-0002-0975-9270'
repository-code: 'https://github.com/RicCampbell/FAIR4RS_repos_dois'
url: 'https://github.com/RicCampbell/FAIR4RS_repos_dois'
abstract: >-
While putting your software online certainly helps it satisfy the FAIR principles, simply doing so might not be enough for other researchers to actually find and utilise what you’ve put out there.
It’s important to know the benefits and issues with where you store and publish your data, and to make the most of the tools these platforms provide, such as Digital Object Identifiers (DOIs). It’s also important to know best practice for how to increase the visibility and citability of your work in cases where your chosen platform lacks these features.
This course will introduce and explore worked examples of elements that you should consider when publishing your software, which will help you easily reference your work, and also help make it more findable and reusable by others.
keywords:
- carpentries
- fair4rs
- university of sheffield
- repositories
- dois
- metadata
- citation
license: CC-BY-4.0
As you can see, this has all the relevant metadata needed for full citation, and you can see where it was created. This has also been updated manually as well when things have changed, but there are lots of places online where you can see the fields needed and the style they should be in if you have a quick search.
If this is inside a GitHub repo, then on the right hand side, you will be able to get the citation text, or download one to be imported into a reference manager, via a BibTeX file.
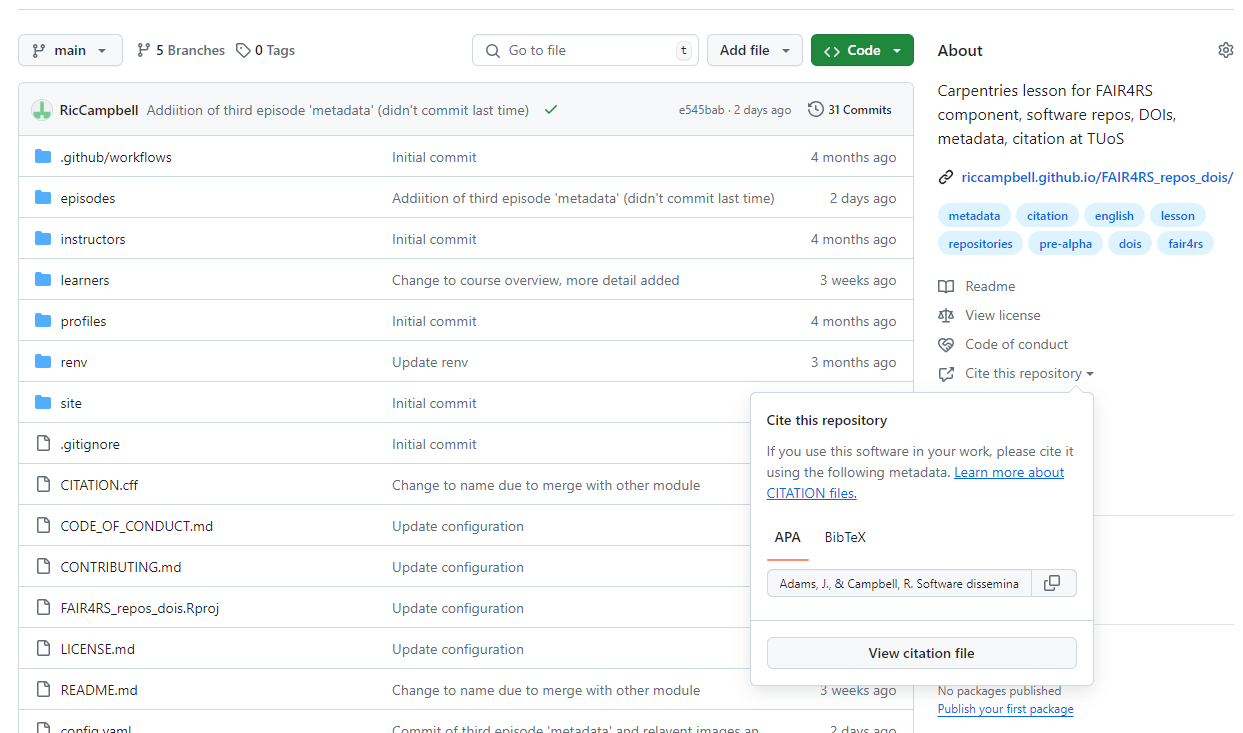
So this is great for people citing your work and for getting the correct information to cite other people’s work.
Not all citations are created equal
GitHub is not the only platform to make use of
CITATION.cff
files; however, not every platform or
repository uses them either. As previously mentioned, you can link your
GitHub repos to ORDA, the University of Sheffield repository, and ORDA
has an easy way of creating citations as well:
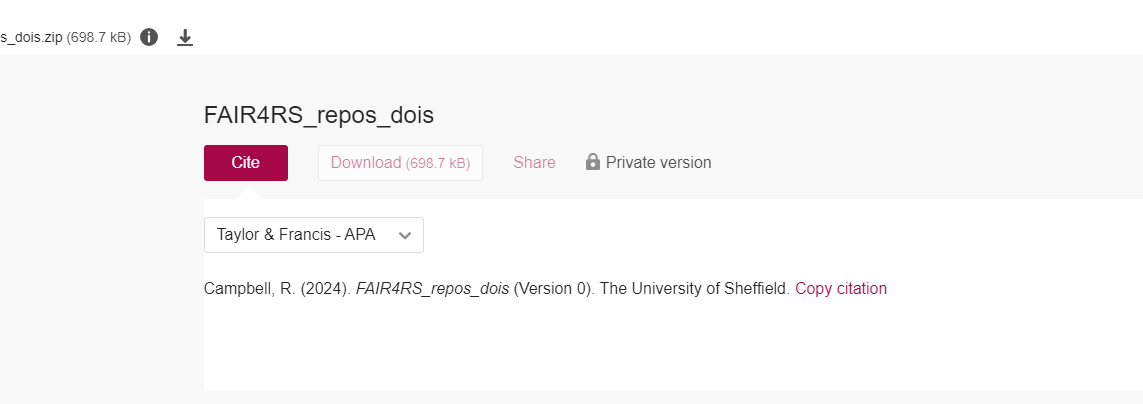
However, the two citations that we’ve just seen for the same output
are not the same. The issue comes with the fact that Figshare (which the
University of Sheffield repository, ORDA, is an instance of), does not
look in the CITATION.cff
file, but instead creates one from
the metadata that is entered for the deposit. In this example, the
author list is different as I’ve entered all authors into the
CITATION.cff
file, but have not edited this in ORDA. It’s
easy to add these, but it means repetition of work. While this isn’t
ideal, it’s important to try to get everything the same where possible.
You don’t know where your work may end up once it’s been made open, so
controlling what you can to start with really improves chances of the
provenance being kept with it, and the ability of people to find the
original repository. The CITATION.cff
file should also
always be kept with your work if it does get shared onwards, and helps
to this end as well.
GitHub citation:
Adams, J., & Campbell, R. Software dissemination and
impact [Computer software]. https://github.com/RicCampbell/FAIR4RS_repos_dois
ORDA citation;
Campbell, R. (2024). FAIR4RS_repos_dois (Version 0).
The University of Sheffield.
Extending your CITATION.cff file
There are also a lot of standardised fields that you can add to your
CITATION.cff
file, other than the ones we have already
seen. You can link to a related journal article if there is one, or you
could cite a dataset that is contained in your GitHub repo. For more
information about these extensions, GitHub provides an easy-to-follow help
page on the subject.
Key Points
- Citation is important to ensure that the correct recognition and credit is assigned to all work
- Citation of software differs from that of articles, but is needed to aid the reproducibility of work
-
CITATION.cff
files should contain all the necessary metadata and help create citations for use. - You may need to complete the relevant fields in multiple places to ensure correct citation in all cases.